Workshop on Empirical Methods in Social Science Research
March 28 and 29, 2025
At this workshop, held at the Phyfer Auditorium in the Wilbur O. and Ann Powers College of Business at Clemson University, leading researchers in econometrics and causal inference made their new methods accessible to applied researchers.
Friday, March 28
"Difference-in-Differences with Heterogenous Treatment Effects"
This presentation highlights how to use causal-inference first principles in Difference-in-difference designs without restricting treatment effect heterogeneity. It starts with a simple two-period design and discusses how to incorporate covariates into the model. It then shows that the same principles can be applied to staggered adoption setups."A Unified Approach to Regression-Based Difference-in-Differences Designs with Staggered Entry"
This talk provides an overview of regression-based difference-in-difference estimators in staggered designs, possibly also with staggered exit. Estimators can be derived in a single framework that imposes no-anticipation and conditional-parallel-trend assumptions on the outcome in the untreated state. These include linear and nonlinear models that are easily estimated using pooled quasi-maximum likelihood methods. The setup clarifies the difference between estimators that aggregate pre-treatment periods as the reference period -so-called "lag only" estimators -and those that use a single pre-treatment period as the reference period -so-called "event study" or "leads and lags" estimators. Simple pre-trend tests that allow full flexibility in the covariates are readily available, and it is straightforward to allow cohort-specific trends that can also depend on control variables. I show how basic Stata estimation commands combined with the margins command make aggregation of effects and standard error calculations straightforward."Estimation and Inference in Boundary Discontinuity Designs"
Boundary discontinuity designs are used to learn about treatment effects along a continuous boundary that splits units into control and treatment groups according to their bivariate score variable. These research designs are also called Multi-Score regression discontinuity designs, a leading special case being Geographic regression discontinuity designs. We study the statistical properties of commonly used local polynomial treatment effects estimators along the continuous treatment assignment boundary. We consider two distinct approaches: one based explicitly on the bivariate score variable for each unit and the other based on their univariate distance to the boundary. For each approach, we present pointwise and uniform estimation and inference methods for the treatment effect function over the assignment boundary. Importantly, we show that methods based on univariate distance to the boundary exhibit an irreducible large misspecification bias when the assignment boundary has kinks or other irregularities, making the distance-based approach unsuitable for empirical work in those settings. In contrast, methods based on the bivariate score variable do not suffer from that drawback. We illustrate our methods with an empirical application and simulations."Inference for Regression with Spatially Correlated Data"
This talk discusses best practice methods, where possible, for inference for regression with data that are correlated across some measure of distance, most often geographic distance. A positive correlation in distance leads to an information loss relative to independent data. Failure to adequately account for this loss in precision leads to standard errors for model parameters that can be greatly underestimated, confidence intervals that are too narrow, and hypothesis tests that greatly over-reject. Currently, many applied studies fail to fully account for this loss in precision. This talk discusses cluster-robust inference, which is appropriate when spatial correlation disappears when a threshold is crossed, and spatial HAC inference, which is appropriate when a spatial correlation dampens in distance. It additionally addresses the common complication that standard asymptotic results can provide a poor approximation in typical sample sizes, presents the recently proposed design-based approach to inference and covers the more structural spatial autoregressive models.Saturday, March 29
"Difference-in-Differences with a Continuous Treatment"
This talk discusses difference-in-differences (DiD) identification strategies when the treatment can take multiple values or is continuous. Part of the talk covers how to extend DiD arguments from a setting with a binary treatment to these more complicated treatment regimes. The other part covers new conceptual issues that arise in settings with a multi-valued/continuous treatment that are not present in settings with a binary treatment."Identification of Treatment Effects with Bunching Designs"
This talk gives an overview of the existing strategies for the identification of causal effects in models with endogeneity, which leverage bunching in the treatment variable. It covers some of the most user-friendly estimators, some strategies for robustness analysis, and a light discussion of the most recent nonparametric methods for the identification of local effects.d adoption setups."Zombie Econometrics: The Linear Probability Specification"
Models of binary outcomes are widely used in many disciplines, including economics and began in the modern literature with the bio-assay studies of Bliss (1934) and Finney (1947). Linear specifications for binary outcomes began to appear in the economics literature in the 1950s, but by the early 1980s, they had been largely abandoned because of conspicuous statistical shortcomings. Nonetheless, a few studies using linear specifications for binary outcomes continued to appear, and with the arrival of the "Credibility Revolution" (e.g., Angrist and Pischke, 2010), recent years have seen thousands of papers featuring linear specifications each year. This phenomenon is, however, confined to the economics literature. This paper examines the numerous econometric shortcomings of the linear specification for binary outcomes and provides a cautionary tale about its use. View slides.Meet Our Speakers
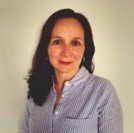
Carolina Caetano is an Assistant Professor of Economics at the University of Georgia. She earned her Ph.D. from UC Berkeley. Her research focuses on econometrics, both in developing methods to improve causal inference and applying them to fields such as health economics and child development. Most of Caetano's work is focused on developing methods for identifying causal effects in settings where standard identification strategies are unavailable or unreliable. Her work has been published in leading journals, including Econometrica, the Journal of Labor Economics and the Journal of Econometrics.
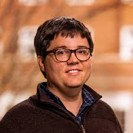
Brantly Callaway is an Associate Professor at the University of Georgia. Callaway's primary research interests are in Microeconometrics. Most of his research is concerned with how to use panel data (repeated observations on the same individual or firm over time) to think about the causal effects of economic policies. Callaway has been particularly interested in developing methods related to understanding how the effects of policies vary across different individuals. Callaway is also interested in applications in labor economics. Specifically, his work has focused on understanding the effects of job displacement, minimum wage policies, intergenerational mobility and unions.
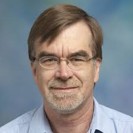
Colin Cameron is a Distinguished Professor of Economics in the Department of Economics at the University of California - Davis, where he teaches econometrics courses at various levels as well as an undergraduate course in health economics. He was educated at the Australian National University (undergraduate) and Stanford University (graduate). He was previously an Assistant Professor at The Ohio State University (1987-89). He has held visiting positions at the University of Sydney, Australian National University, University of New South Wales and Indiana University - Bloomington. He is a Fellow of the International Association for Applied Econometrics and a Senior Fellow of the Rimini Centre for Economic Analysis. Cameron's main research is in micro econometrics for cross-section data and has appeared in many of the leading all-around economics journals and econometrics field journals. His earlier research was on count data modeling, which he did jointly with Pravin K. Trivedi. His more recent research is on cluster-robust inference and includes the 2008 Review of Economics and Statistics article "Bootstrap-based Improvements for Inference with Clustered Errors," the 2011 Journal of Business and Statistics article "Robust Inference with Multi-Way Clustering" (both joint work with Jonah Gelbach and Doug Miller) and the 2015 Journal of Human Resources article "A Practitioner's Guide to Cluster-Robust Inference" (joint with Doug Miller). He is the co-author of Pravin Trivedi's books "Regression Analysis with Count Data, Microeconometrics: Methods and Applications" and "Microeconometrics using Stata."
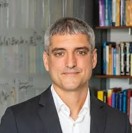
Matias Cattaneo is a Professor of Operations Research and Financial Engineering (ORFE) at Princeton University, where he is also an Associated Faculty in the School of Public and International Affairs (SPIA), the Department of Economics, and the Program in Latin American Studies (PLAS), and an Affiliated Faculty in the Data-Driven Social Science (DDSS) initiative, the AI at Princeton initiative, and the Center for Statistics and Machine Learning (CSML). His research spans econometrics, statistics, data science and decision science, with applications to program evaluation and causal inference. Most of his work is interdisciplinary and motivated by quantitative problems in the social, behavioral and biomedical sciences. As part of his main research agenda, he has developed novel nonparametric, semiparametric, high-dimensional and machine-learning estimation and inference procedures with demonstrably superior robustness.
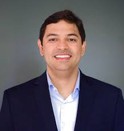
Pedro Sant'Anna is an Associate Professor of Economics at Emory University and an Amazon Visiting Academic. Sant'Anna is a passionate applied econometrician, working in causal inference and semi- and non-parametric methods. Much of his recent work aims to develop, better understand, and further improve Difference-in-Differences (DiD) methods. Sant'Anna has published in top journals in economics and has given guest lectures and seminars on DiD topics at leading universities around the world. He is also a co-author of several open-source packages for DiD methods, allowing him to have first-hand experience with the practicalities of these modern tools. His industry experience also allows him to effectively communicate with a broad audience.
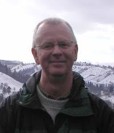
Paul W. Wilson is the J. Wilson Newman Professor of Economics and Professor of Computer Science at Clemson University, where he has worked since 2006. Wilson's research encompasses nonparametric estimation and inference, mathematical and computational statistics and empirical microeconomics. Wilson is a frequent visitor at the Federal Reserve Bank of St. Louis and the Institut de Statistique, Biostatistique et Sciences Actuarielles at Universite Catholiquede Louvain in Louvain-la-Neuve, Belgium. He earned his Ph.D. from Brown University, and prior to his appointment at Clemson, he served on the faculty at both the University of Texas at Austin and the University of Georgia.
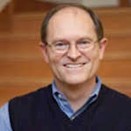
Jeffrey Wooldridge is a University Distinguished Professor of Economics at Michigan State University, where he has taught since 1991. From 1986 to 1991, he served as Assistant Professor of Economics at the Massachusetts Institute of Technology (MIT). Wooldridge has published more than 70 articles in internationally recognized journals, as well as several chapters in well-respected books. He is also the author of "Econometric Analysis of Cross Section and Panel Data." His work has earned numerous awards, including the Alfred P. Sloan Research Fellowship, the Plura Scripsit Award for Econometric Theory, the Sir Richard Stone Prize from the Journal of Applied Econometrics and three graduate teacher-of-the-year awards from MIT. A fellow of the Econometric Society, the Journal of Econometrics and the International Association for Applied Econometrics, Wooldridge has been editor of the Journal of Business and Economic Statistics and Econometrics and co-editor of "Economics Letters." He has also served on the editorial boards of the Journal of Econometrics, Econometric Theory and the Review of Economics and Statistics. Wooldridge received his B.A. with majors in computer science and economics from the University of California, Berkeley, and received his Ph.D. in economics from the University of California, San Diego.